Navigating the Ethical Challenges of Algorithmic Decision-Making
You prefer to listen to this article? Click here to access our AI-generated audio version:
Navigating the Ethical Challenges of Algorithmic Decision-Making
Navigating the Ethical Challenges of Algorithmic Decision-Making
Artificial Intelligence (AI) is transforming our world, from personalized recommendations to advanced medical diagnostics. However, as AI becomes more integrated into our lives, a critical issue has emerged: algorithmic bias. This bias occurs when AI systems are trained on flawed or incomplete data that lacks diversity, leading to unfair or discriminatory outcomes and often perpetuating existing inequalities.
What is AI Bias?
AI bias arises when algorithms reflect and amplify societal prejudices embedded in their training data. For example, suppose an AI system is trained predominantly on data from one demographic group. In that case, it may perform poorly for others, leading to unfair decisions in areas like hiring, healthcare, and law enforcement.
The Dual Nature of AI
AI offers immense benefits, but these are accompanied by significant risks. In healthcare, for instance, AI can assist in diagnosing diseases, but if the training data lacks diversity, the AI may misdiagnose conditions in underrepresented groups, exacerbating health disparities.
Global Implications: Data Colonialism
AI bias isn’t just a local issue—it has global ramifications. In many cases, data from the Global South is used to train AI systems that benefit the Global North, a practice known as data colonialism. This exploitation deepens global inequalities, as those in the Global South often do not share in the benefits of AI technologies.
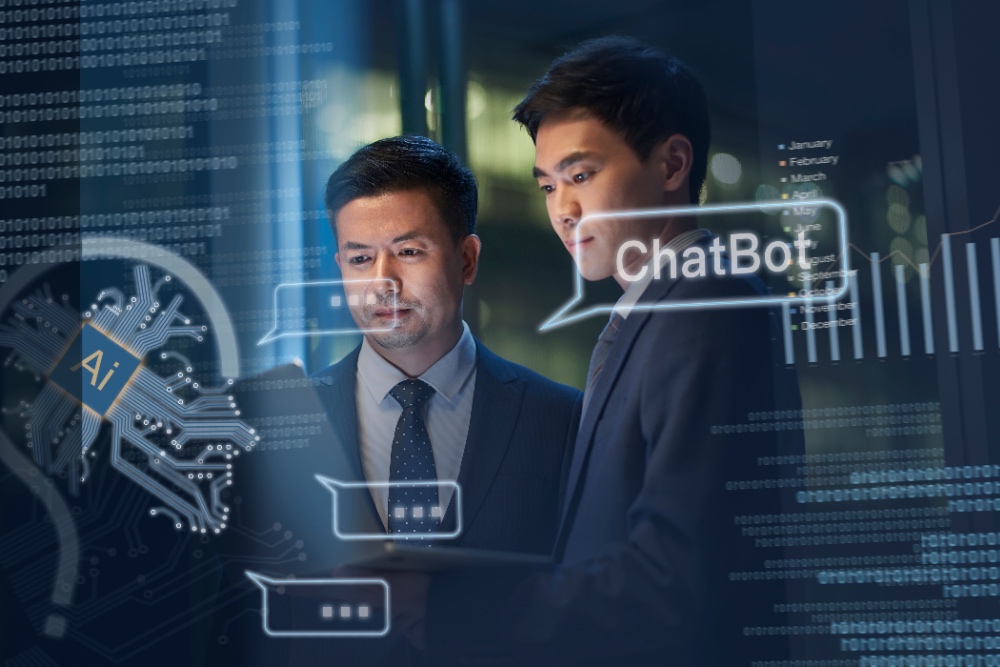
Addressing AI Bias
To mitigate AI bias, it is essential to adopt a responsible approach to AI development. This involves recognizing the duality of AI—its potential for both benefit and harm—and taking proactive steps to minimize risks. Here are some key strategies:
- Diverse and Representative Data: Ensuring that AI systems are trained on diverse and representative datasets is crucial. This includes data from different demographic groups, geographic regions, and socio-economic backgrounds to minimize bias.
- Transparency and Accountability: AI systems should be transparent, with clear explanations of how decisions are made. Additionally, there should be mechanisms for accountability, allowing users to challenge and understand decisions that negatively impact them.
- Ethics by Design: Integrating ethical considerations into the design and development of AI systems is vital. This includes ongoing assessments of how AI systems impact different groups and the potential risks involved.
- Global Collaboration: Addressing AI bias requires global collaboration, with input from diverse stakeholders, including those from marginalized communities. Policymakers, industry leaders, and civil society must work together to create regulations and standards that ensure AI development is fair and inclusive.
Conclusion: A Path Forward
As we move forward into an increasingly AI-driven world, it is crucial to remain vigilant about the biases embedded in these technologies. Only by acknowledging and addressing these issues can companies ensure that AI serves as a tool for positive change, rather than perpetuating existing inequalities.
Murikah, W., Nthenge, J. K., & Musyoka, F. M. (2024). Bias and ethics of AI systems applied in auditing – A systematic review. Scientific African, 16, e02281. https://doi.org/10.1016/j.sciaf.2024.e02281
Ukanwa, K. (2024). Algorithmic bias: Social science research integration through the 3-D Dependable AI Framework. Current Opinion in Psychology, 58, 101836. https://doi.org/10.1016/j.copsyc.2024.101836
Caro-Burnett, J., & Kaneko, S. (2022). Is society ready for AI ethical decision-making? Lessons from a study on autonomous cars. Journal of Behavioral and Experimental Economics, 98, 101881. https://doi.org/10.1016/j.socec.2022.101881
Arora, A., Barrett, M., Lee, E., Oborn, E., & Prince, K. (2023). Risk and the future of AI: Algorithmic bias, data colonialism, and marginalization. Information and Organization, 33(3), 100478. https://doi.org/10.1016/j.infoandorg.2023.100478
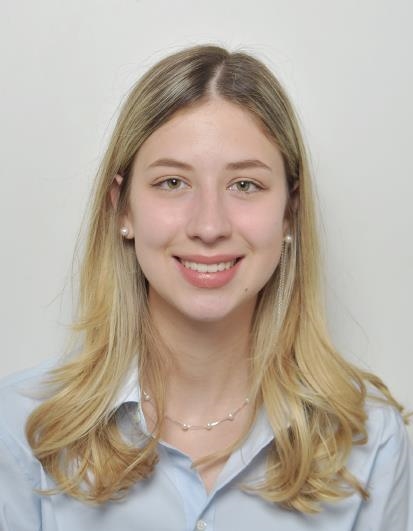
Raphaela Pouzar
Marketing Team Assistant
Raphaela Pouzar, a Bachelor of Business Administration student at IMC Krems, works at MDI as a marketing assistant alongside her studies. Additionally, she is currently pursuing an AI certificate from Harvard Business School.
Download our International Whitepaper: